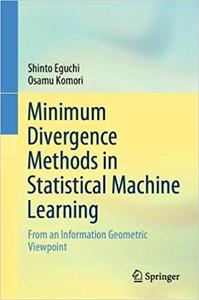
Minimum Divergence Methods in Statistical Machine Learning: From an Information Geometric Viewpoint
English | 2022 | ISBN: 4431569200 | 231 Pages | PDF EPUB | 22 MB
This book explores minimum divergence methods of statistical machine learning for estimation, regression, prediction, and so forth, in which we engage in information geometry to elucidate their intrinsic properties of the corresponding loss functions, learning algorithms, and statistical models. One of the most elementary examples is Gauss's least squares estimator in a linear regression model, in which the estimator is given by minimization of the sum of squares between a response vector and a vector of the linear subspace hulled by explanatory vectors. This is extended to Fisher's maximum likelihood estimator (MLE) for an exponential model, in which the estimator is provided by minimization of the Kullback-Leibler (KL) divergence between a data distribution and a parametric distribution of the exponential model in an empirical analogue. Thus, we envisage a geometric interpretation of such minimization procedures such that a right triangle is kept with Pythagorean identity in the sense of the KL divergence. This understanding sublimates a dualistic interplay between a statistical estimation and model, which requires dual geodesic paths, called m-geodesic and e-geodesic paths, in a framework of information geometry.
Buy Premium In Link Below To Support
https://hot4share.com/pfi6ue4uumjw/crkpi.Minimum.Divergence.Methods.in.Statistical.Machine.Learning.rar.html

https://rapidgator.net/file/91d2734152422ff1fc5357c0b5051333/crkpi.Minimum.Divergence.Methods.in.Statistical.Machine.Learning.rar.html

https://uploadgig.com/file/download/C0ec78c4b4Ac0530/crkpi.Minimum.Divergence.Methods.in.Statistical.Machine.Learning.rar

https://nitro.download/view/E4CB7CE4C3E5724/crkpi.Minimum.Divergence.Methods.in.Statistical.Machine.Learning.rar