Free Ebooks Download :
Machine Learning from Weak Supervision An Empirical Risk Minimization Approach (The MIT Press)
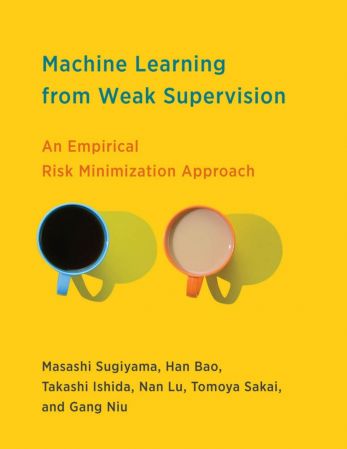
English | 2022 | ISBN: 0262047071 | 325 pages | PDF | 37.05 MB
Fundamental theory and practical algorithms of weakly supervised classification, emphasizing an approach based on empirical risk minimization.
Standard machine learning techniques require large amounts of labeled data to work well. When we apply machine learning to problems in the physical world, however, it is extremely difficult to collect such quantities of labeled data. In this book Masashi Sugiyama, Han Bao, Takashi Ishida, Nan Lu, Tomoya Sakai and Gang Niu present theory and algorithms for weakly supervised learning, a paradigm of machine learning from weakly labeled data. Emphasizing an approach based on empirical risk minimization and drawing on state-of-the-art research in weakly supervised learning, the book provides both the fundamentals of the field and the advanced mathematical theories underlying them. It can be used as a reference for practitioners and researchers and in the classroom.
The book first mathematically formulates classification problems, defines common notations, and reviews various algorithms for supervised binary and multiclass classification. It then explores problems of binary weakly supervised classification, including positive-unlabeled (PU) classification, positive-negative-unlabeled (PNU) classification, and unlabeled-unlabeled (UU) classification. It then turns to multiclass classification, discussing complementary-label (CL) classification and partial-label (PL) classification. Finally, the book addresses more advanced issues, including a family of correction methods to improve the generalization performance of weakly supervised learning and the problem of class-prior estimation.
Links are Interchangeable - No Password - Single Extraction
Machine Learning from Weak Supervision An Empirical Risk Minimization Approach (The MIT Press) Fast Download
Machine Learning from Weak Supervision An Empirical Risk Minimization Approach (The MIT Press) Full Download
free Machine Learning from Weak Supervision An Empirical Risk Minimization Approach (The MIT Press), Downloads Machine Learning from Weak Supervision An Empirical Risk Minimization Approach (The MIT Press), Rapidgator Machine Learning from Weak Supervision An Empirical Risk Minimization Approach (The MIT Press), Nitroflare Machine Learning from Weak Supervision An Empirical Risk Minimization Approach (The MIT Press), Mediafire Machine Learning from Weak Supervision An Empirical Risk Minimization Approach (The MIT Press), Uploadgig Machine Learning from Weak Supervision An Empirical Risk Minimization Approach (The MIT Press), Mega Machine Learning from Weak Supervision An Empirical Risk Minimization Approach (The MIT Press), Torrent Download Machine Learning from Weak Supervision An Empirical Risk Minimization Approach (The MIT Press), HitFile Machine Learning from Weak Supervision An Empirical Risk Minimization Approach (The MIT Press) , GoogleDrive Machine Learning from Weak Supervision An Empirical Risk Minimization Approach (The MIT Press),
Please feel free to post your Machine Learning from Weak Supervision An Empirical Risk Minimization Approach (The MIT Press) Download, Tutorials, Ebook, Audio Books, Magazines, Software, Mp3, Free WSO Download , Free Courses Graphics , video, subtitle, sample, torrent, NFO, Crack, Patch,Rapidgator, mediafire,Mega, Serial, keygen, Watch online, requirements or whatever-related comments here.