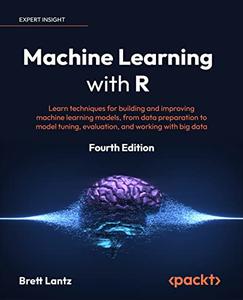
Free Download Machine Learning with R
by Lantz, Brett;
English | 2023 | ISBN: 1801071322 | 763 pages | True PDF | 43.78 MB
Learn how to solve real-world data problems using machine learning and R
Purchase of the print or Kindle book includes a free eBook in PDF format.
Key Features
The 10th Anniversary Edition of the bestselling R machine learning book, updated with 50% new content for R 4.0.0 and beyondHarness the power of R to build flexible, effective, and transparent machine learning modelsLearn quickly with this clear, hands-on guide by machine learning expert Brett Lantz
Book Description
Machine learning, at its core, is concerned with transforming data into actionable knowledge. R offers a powerful set of machine learning methods to quickly and easily gain insight from your data.
Machine Learning with R, Fourth Edition, provides a hands-on, accessible, and readable guide to applying machine learning to real-world problems. Whether you are an experienced R user or new to the language, Brett Lantz teaches you everything you need to know for data pre-processing, uncovering key insights, making new predictions, and visualizing your findings. This 10th Anniversary Edition features several new chapters that reflect the progress of machine learning in the last few years and help you build your data science skills and tackle more challenging problems, including making successful machine learning models and advanced data preparation, building better learners, and making use of big data.
You'll also find this classic R data science book updated to R 4.0.0 with newer and better libraries, advice on ethical and bias issues in machine learning, and an introduction to deep learning. Whether you're looking to take your first steps with R for machine learning or making sure your skills and knowledge are up to date, this is an unmissable read that will help you find powerful new insights in your data.
What you will learn
Learn the end-to-end process of machine learning from raw data to implementationClassify important outcomes using nearest neighbor and Bayesian methodsPredict future events using decision trees, rules, and support vector machinesForecast numeric data and estimate financial values using regression methodsModel complex processes with artificial neural networksPrepare, transform, and clean data using the tidyverseEvaluate your models and improve their performanceConnect R to SQL databases and emerging big data technologies such as Spark, Hadoop, H2O, and TensorFlow
Who this book is for
This book is designed to help data scientists, actuaries, data analysts, financial analysts, social scientists, business and machine learning students, and any other practitioners who want a clear, accessible guide to machine learning with R. No R experience is required, although prior exposure to statistics and programming is helpful.
Table of Contents
Introducing Machine LearningManaging and Understanding DataLazy Learning - Classification Using Nearest NeighborsProbabilistic Learning - Classification Using Naive BayesDivide and Conquer - Classification Using Decision Trees and RulesForecasting Numeric Data - Regression MethodsBlack-Box Methods - Neural Networks and Support Vector MachinesFinding Patterns - Market Basket Analysis Using Association RulesFinding Groups of Data - Clustering with k-meansEvaluating Model PerformanceBeing Successful with Machine LearningAdvanced Data PreparationChallenging Data - Too Much, Too Little, Too ComplexBuilding Better LearnersMaking Use of Big Data