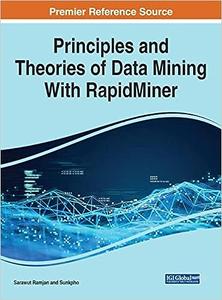
Free Download Principles and Theories of Data Mining With RapidMiner
by Ramjan Sarawut
English | 2023 | ISBN: 1668447312 | 326 pages | True PDF EPUB | 35.04 MB
The demand for skilled data scientists is rapidly increasing as more organizations recognize the value of data-driven decision- making. Data science, data management, and data mining are all critical components for various types of organizations, including large and small corporations, academic institutions, and government entities. For companies, these components serve to extract insights and value from their data, empowering them to make evidence-driven decisions and gain a competitive advantage by discovering patterns and trends and avoiding costly mistakes. Academic institutions utilize these tools to analyze large datasets and gain insights into various scientific fields of study, including genetic data, climate data, financial data, and in the social sciences they are used to analyze survey data, behavioral data, and public opinion data. Governments use data science to analyze data that can inform policy decisions, such as identifying areas with high crime rates, determining which regions need infrastructure development, and predicting disease outbreaks. However, individuals who are not data science experts, but are experts within their own fields, may need to apply their experience to the data they must manage, but still struggle to expand their knowledge of how to use data mining tools such as RapidMiner software. Principles and Theories of Data Mining With RapidMiner is a comprehensive guide for students and individuals interested in experimenting with data mining using RapidMiner software. This book takes a practical approach to learning through the RapidMiner tool, with exercises and case studies that demonstrate how to apply data mining techniques to real-world scenarios. Readers will learn essential concepts related to data mining, such as supervised learning, unsupervised learning, association rule mining, categorical data, continuous data, and data quality. Additionally, readers will learn how to apply data mining techniques to popular algorithms, including k-nearest neighbor (K-NN), decision tree, naïve bayes, artificial neural network (ANN), k-means clustering, and probabilistic methods. By the end of the book, readers will have the skills and confidence to use RapidMiner software effectively and efficiently, making it an ideal resource for anyone, whether a student or a professional, who needs to expand their knowledge of data mining with RapidMiner software.