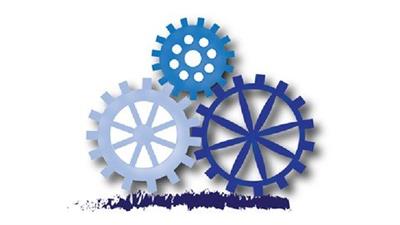
Genre: eLearning | MP4 | Video: h264, 1280x720 | Audio: aac, 44100 Hz
Language: English | VTT | Size: 1.50 GB | Duration: 12 sections | 69 lectures | (4h 25m)
What you'll learn
Learn about filter, embedded and wrapper methods for feature selection
Find out about hybdrid methods for feature selection
Select features with Lasso and decision trees
Implement different methods of feature selection with Python
Learn why less (features) is more
Reduce the feature space in a dataset
Build simpler, faster and more reliable machine learning models
Analyse and understand the selected features
Discover feature selection techniques used in data science competitions
Requirements
A Python installation
Jupyter notebook installation
Python coding skills
Some experience with Numpy and Pandas
Familiarity with Machine Learning algorithms
Familiarity with scikit-learn
Description
Welcome to Feature Selection for Machine Learning, the most comprehensive course on feature selection available online.
In this course, you will learn how to select the variables in your data set and build simpler, faster, more reliable and more interpretable machine learning models.
Who is this course for?
You've given your first steps into data science, you know the most commonly used machine learning models, you probably built a few linear regression or decision tree based models. You are familiar with data pre-processing techniques like removing missing data, transforming variables, encoding categorical variables. At this stage you've probably realized that many data sets contain an enormous amount of features, and some of them are identical or very similar, some of them are not predictive at all, and for some others it is harder to say.
You wonder how you can go about to find the most predictive features. Which ones are OK to keep and which ones could you do without? You also wonder how to code the methods in a professional manner. Probably you did your online search and found out that there is not much around there about feature selection. So you start to wonder: how are things really done in tech companies?
This course will help you! This is the most comprehensive online course in variable selection. You will learn a huge variety of feature selection procedures used worldwide in different organizations and in data science competitions, to select the most predictive features.
What will you learn?
I have put together a fantastic collection of feature selection techniques, based on scientific articles, data science competitions and of course my own experience as a data scientist.
Specifically, you will learn:
How to remove features with low variance
How to identify redundant features
How to select features based on statistical tests
How to select features based on changes in model performance
How to find predictive features based on importance attributed by models
How to code procedures elegantly and in a professional manner
How to leverage the power of existing Python libraries for feature selection
Throughout the course, you are going to learn multiple techniques for each of the mentioned tasks, and you will learn to implement these techniques in an elegant, efficient, and professional manner, using Python, Scikit-learn, pandas and mlxtend.
At the end of the course, you will have a variety of tools to select and compare different feature subsets and identify the ones that returns the simplest, yet most predictive machine learning model. This will allow you to minimize the time to put your predictive models into production.
This comprehensive feature selection course includes about 70 lectures spanning ~8 hours of video, and ALL topics include hands-on Python code examples which you can use for reference and for practice, and re-use in your own projects.
In addition, I update the course regularly, to keep up with the Python libraries new releases and include new techniques when they appear.
So what are you waiting for? Enroll today, embrace the power of feature selection and build simpler, faster and more reliable machine learning models.
Who this course is for:
Beginner Data Scientists who want to understand how to select variables for machine learning
Intermediate Data Scientists who want to level up their experience in feature selection for machine learning
Advanced Data Scientists who want to discover alternative methods for feature selection
Software engineers and academics switching careers into data science
Software engineers and academics stepping into data science
Data analysts who want to level up their skills in data science
Buy Premium From My Links To Get Resumable Support,Max Speed & Support Me

https://uploadgig.com/file/download/9c24c6A884346D6F/4i4x7.Feature.Selection.for.Machine.Learning.part1.rar
https://uploadgig.com/file/download/5cf0D488760256c3/4i4x7.Feature.Selection.for.Machine.Learning.part2.rar

https://rapidgator.net/file/4ffbe177ce136b770b2282c85c07e096/4i4x7.Feature.Selection.for.Machine.Learning.part1.rar.html
https://rapidgator.net/file/6b7795602e2aec6a7ee67e273940a285/4i4x7.Feature.Selection.for.Machine.Learning.part2.rar.html

http://nitroflare.com/view/0A16F3896EAB26D/4i4x7.Feature.Selection.for.Machine.Learning.part1.rar
http://nitroflare.com/view/E09DEEB9E12A953/4i4x7.Feature.Selection.for.Machine.Learning.part2.rar