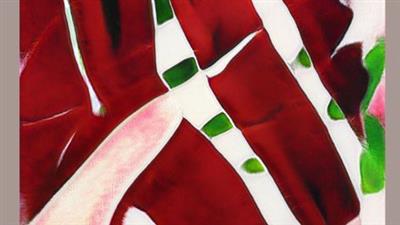
MP4 | Video: h264, 1280x720 | Audio: AAC, 44.1 KHz
Language: English | Size: 1.58 GB | Duration: 3h 26m
What you'll learn
Deep Learning for Natural Language Processing
Sentence Embeddings: Bag of words, Doc2Vec, SkipThought, InferSent, DSSM, USE, MTDNN, SentenceBERT
Generative Transformer Models: UniLM, Transformer-XL and XLNet, MASS, BART, CTRL, T5, ProphetNet
DL for NLP
Requirements
Basics of machine learning
Recurrent Models: RNNs, LSTMs, GRUs and variants
Basic understanding of Transformer based models and word embeddings
Description
This course is a part of "Deep Learning for NLP" Series. In this course, I will introduce concepts like Sentence embeddings and Generative Transformer Models. These concepts form the base for good understanding of advanced deep learning models for modern Natural Language Generation.
The course consists of two main sections as follows.
In the first section, I will talk about sentence embeddings. We will start with basic bag of words methods where sentence embedddings are obtained using an aggregation over word embeddings of constituent words. We will talk about averaged bag of words, word mover's distance, SIF and Power means method. Then we will discuss two unsupervised methods: Doc2Vec and SkipThought. Further, we will discuss about supervised sentence embedding methods like recursive neural networks, deep averaging networks and InferSent. CNNs can also be used for computing semantic similarity between two text strings; we will talk about DSSMs for the same. We will also discuss 3 multi-task learning methods including Universal Sentence Encodings and MT-DNN. Lastly, I will talk about SentenceBERT.
In the second section, I will talk about multiple Generative Transformer Models. We will start with UniLM. Then we will talk about segment recurrence and relative position embeddings in Transformer-XL. Then get to XLNets which use Transformer-XL along with permutation language modeling. Next we will understand span masking in MASS and also discuss various noising methods on BART. We will then discuss about controlled natural language generation using CTRL. We will discuss how T5 models every learning task as a text-to-text task. Finally, we will discuss how ProphetNet extends 2-stream attention modeling from XLNet to n-stream attention modeling, thereby enabling n-gram predictions.
Who this course is for:
Beginners in deep learning
Python developers interested in data science concepts
Masters or PhD students who wish to learn deep learning concepts quickly
Homepage
https://www.udemy.com/course/ahol-dl4nlp3/
Buy Premium From My Links To Get Resumable Support,Max Speed & Support Me

https://uploadgig.com/file/download/C9983f3F077ee012/wmam5.Deep.Learning.for.NLP..Part.3.part1.rar
https://uploadgig.com/file/download/106E9a3eA7044477/wmam5.Deep.Learning.for.NLP..Part.3.part2.rar

https://rapidgator.net/file/80103379f5624c48e6dd7bf48953110a/wmam5.Deep.Learning.for.NLP..Part.3.part1.rar.html
https://rapidgator.net/file/89265525ae1e82096b0c69ec1ae1d6fd/wmam5.Deep.Learning.for.NLP..Part.3.part2.rar.html

http://nitro.download/view/1101DB73D713648/wmam5.Deep.Learning.for.NLP..Part.3.part1.rar
http://nitro.download/view/1C08723AB93FDB2/wmam5.Deep.Learning.for.NLP..Part.3.part2.rar